EECS Seminar: Reinforcement Learning with Robustness and Safety Guarantees
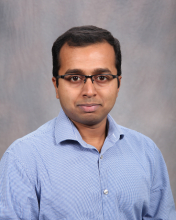
Assistant Professor
Department of Electrical and Computer Engineering
Texas A&M University
Abstract: Reinforcement Learning (RL) is the class of machine learning that addresses the problem of learning to control unknown dynamical systems. RL has achieved remarkable success recently in applications like playing games and robotics. However, most of these successes are limited to very structured or simulated environments. When applied to real-world systems, RL algorithms face two fundamental sources of fragility. First, the real-world system parameters can be very different from that of the nominal values used for training RL algorithms. Second, the control policy for any real-world system is required to maintain some necessary safety criteria to avoid undesirable outcomes. Most deep RL algorithms overlook these fundamental challenges which often results in learned policies that perform poorly in the real-world settings. In this talk, I will present two approaches to overcome these challenges. First, I will present an RL algorithm that is robust against the parameter mismatches between the simulation system and the real-world system. Second, I will discuss a safe RL algorithm to learn policies such that the frequency of visiting undesirable states and expensive actions satisfies the safety constraints. I will also briefly discuss some practical challenges due to the sparse reward feedback and the need for rapid real-time adaptation in real-world systems, and the approaches to overcome these challenges.
Bio: Dileep Kalathil is anassistant professor in the Department of Electrical and Computer Engineering at Texas A&M University (TAMU). His main research area is reinforcement learning theory and algorithms, their applications in mobile robotics and communication networks and power systems. Before joining TAMU, he was a postdoctoral researcher in the EECS department at UC Berkeley. He received his Ph.D. from University of Southern California (USC) in 2014, where he won the best Ph.D. Dissertation Prize in the Department of Electrical Engineering. He received his M.Tech. from IIT Madras, where he won the award for the best academic performance in the Electrical Engineering Department. He received the NSF CRII Award in 2019 and the NSF CAREER award in 2021. He has been serving as an area chair for the top AI/ML conferences (NeurIPS, ICML, AISTATS) and top control systems conferences (L4DC, IEEE CDC, ACC). He received the Holleran-Bowman Endowed Faculty Fellow Award from the TAMU College of Engineering in 2023. He is a senior member of IEEE.